Measuring and modeling the motor system with machine learning |
| |
Affiliation: | EPFL, Swiss Federal Institute of Technology, Lausanne, Switzerland |
| |
Abstract: | 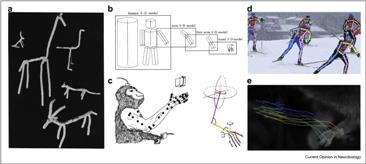 The utility of machine learning in understanding the motor system is promising a revolution in how to collect, measure, and analyze data. The field of movement science already elegantly incorporates theory and engineering principles to guide experimental work, and in this review we discuss the growing use of machine learning: from pose estimation, kinematic analyses, dimensionality reduction, and closed-loop feedback, to its use in understanding neural correlates and untangling sensorimotor systems. We also give our perspective on new avenues, where markerless motion capture combined with biomechanical modeling and neural networks could be a new platform for hypothesis-driven research. |
| |
Keywords: | |
本文献已被 ScienceDirect 等数据库收录! |
|